Predicting In-Hospital Mortality for Traumatic Brain Injury
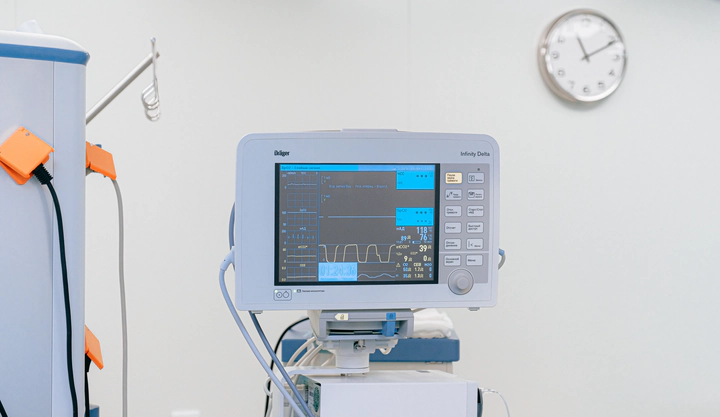
The following is an overview of an independent project of mine. These findings have not been peer reviewed.
Objective
Traumatic Brain Injury (TBI) is a significant health concern that results in disability and death of thousands of people each year in the United States. TBI occurs when a sudden trauma causes damage to the brain and is characterized by a disruption in the normal function of the brain. Symptoms can be mild, moderate, or severe depending on the intensity of damage to the brain tissue. This aim of this analysis was to predict in-hospital mortality in TBI patients with diagnosis of intracranial hemorrhage (ICH), a common and severe feature of severe TBI. Identifying modifiable risk factors and predicting outcomes among ICH patients may be useful in managing prognostic expectations, making treatment decisions, reducing in-hospital mortality, and improving health outcomes.
Methods
Statewide hospital trauma registry data from years 2012 to 2016 were obtained and relevant demographic, injury, and clinical variables were selected for this analysis. The dataset was filtered by ICD-9 codes and other clinical variables to identify patients admitted with traumatic brain injury and intracranial hemorrhage. Patients under the age of 20 were excluded. Missing values were imputed or dropped, and relevant variables were transformed (e.g., encoding, scaling) and engineered as necessary. The dataset was then randomly split with a 75:25 ratio into training and test sets, totaling 8,215 and 2,738 observations, respectively.
Logistic regression, random forest, and gradient boosting models were investigated. For logistic regression, LASSO was used for feature selection, and cubic splines were used for non-linearly associated continuous predictors. For random forest and boosting, all initially selected variables were included in the models. Hyperperamaters were tuned using stratified 5-fold cross-validation in order to find the optimal values. To assess and compare predictive performance for each model on the test set, accuracy, area under the curve (AUC), sensitivity, and specificity were computed. Receiver Operator Curves (ROC) were constructed to illustrate predictive performance. In addition, model performance was assessed and compared after using a downsampling technique on the training set in an attempt to correct the bias introduced by a class imbalance.
Findings
Following variable selection, the final model for logistic regression included gender, age, systolic blood pressure, ICU length of stay, primary payment method, Glasgow coma score (GCS), injury severity score (ISS), and concussion. Significant interaction terms between ICU stay and ISS were included. For random forest and boosting models, all initially selected variables were included in the model. The most important predictors were ISS, GCS, and ICU stay based on feature importance scores. Performance metrics for each of the three models are shown below in Table 1.
Overall, logistic regression outperformed both random forest and gradient boosting models across both data sets. All models had high predictive performance with the full data set, but logistic regression performed slightly better (AUC = 0.923) compared to gradient boosting (AUC = 0.903) and random forest (AUC = 0.886). However, all models had poor sensitivity (0.41-0.462). Considering the potential clinical application of rapid identification of high-risk patients, high sensitivity of the prediction model may be of greater importance to a clinician.
With the downsampled data set, all models saw an increase in AUC and sensitivity and a small decrease in specificity. Logistic regression (AUC = 0.934) once again outperform boosting (AUC = 0.912) and random forest (AUC = 0.914) in terms of AUC. However, the gradient boosting model had the greatest sensitivity (SN = 0.904) among models.
Significance
Real-world data, such as those from trauma registries, provide a rich source of data for training predictive and prognostic models and can be used to make real-world impact in healthcare decision making. Early prediction of in-hospital mortality can help clinicians and other healthcare personnel manage prognostic expectations, inform resource management, make treatment decisions in a timely manner, and ensure that patients are receiving appropriate care.